In this article, we shed light on why the adoption of AI is somehow inhibited. Next, we’ll discuss four phases of AI adoption that can be distinguished and the current state of AI adoption in the market. Finally, we’ll shed light on how you, as an organisation, can move from one adoption phase to the next.
Complexity and the war for talent inhibit AI adoption
Recent research by Gartner pointed out that 33% of technology and service providers with AI technology would invest $1 million or more in these technologies in the next two years. Why? They recognise its potential. Not only to retrieve critical data to improve business efficiency but also to create new products and services, acquire more customers and generate more revenue.
Unfortunately, the adoption of AI hasn’t been going as fast as people and organisations thought it would. The operationalisation of AI still is perceived as being a major challenge. This has to do with the increasing speed of new technology releases (e.g. cloud and MLOps solutions) and their inherent complexity, technological immaturity and a lack of necessary skills among employees. Research by O’Reilly published in March this year[2] underlines the latter, showing a strikingly similar lack of skills, as was the case last year. The research shows it’s not just simply a lack of skills among employees. Recruiting staff to fill the required jobs is still proving to be challenging, giving rise to a true “war for talent”.
When differentiating between organisations that have already operationalised AI and those that experiment and evaluate it, there’s a nuance. Those that are experimenting with and evaluating AI are more likely to state that company culture and identifying appropriate use cases create a bottleneck. It’s no great surprise if you already put some AI into production, is it, as you’re likely to have had a real use case and will already have overcome several cultural challenges.
Embedding AI into an organisation is a journey in itself. Every organisation is unique and so are the challenges faced when making AI an integral component of the business. When zooming out, however, distinct phases of AI adoption can be identified.
Phases of AI adoption
We have identified four distinct phases of AI adoption, based on Gartner’s AI Maturity Model. Practically speaking, this model considers the evolution of AI strategy as a technological and cultural process transformation. The more you proceed through this evolution, the more business value will be unlocked through the adoption of AI (see Figure 1). Note, however, that the process is non-linear and the extent to which you can unlock business value is likely to be more exponential with some ups and downs.
As mentioned, the DDMA AI Maturity Model (Figure 1) distinguishes four phases.
- AI as experiments: this first phase is a highly experimental phase where AI initiatives are likely to be conducted in silos (lab environments) with no central coordination. When you’re in this phase, you’ll probably recognise that people working on AI are a small group of highly skilled individuals (i.e. data scientists, engineers and developers).
- AI in production: although AI in this phase is mainly pushed from the AI group, the business pull is slowly taking off. In terms of organisation, AI working groups are most often centralized and known as AI Centres of Excellence. The AI implementations that exist are mostly brownfield implementations (automating existing processes) rather than greenfield implementations (redesigning processes through the lens of AI possibilities). Data and model pipelines are generally standardised according to the principle of MLOps.
- AI is pervasively used in processes: when arriving in this phase you’ve been through or still going through the process in which technological and cultural transformation intersect. It’s the process in which all personas (business, AI, data, IT) are engaged in the use case lifecycle. AI becomes a key pillar in business strategy and culture, with the strategy being translated into supporting data and AI initiatives. AI implementations are mostly still brownfield rather than greenfield implementations.
Niek de Win (KPN) also points out the importance of an AI-minded company culture:
“After laying the technology foundation required to make an impact with AI, we quickly realised that transforming culture and business processes are the key drivers to delivering AI business value. AI is a paradigm-shifting technology that requires redesigning our digital processes and how we define work.” - AI in business DNA: the last phase is characterised by the fact that processes are redesigned from the ground up (greenfield) through the lens of AI possibilities. Not only are processes improved through AI possibilities, but new products and services are also unlocked thanks to the possibilities provided by AI.
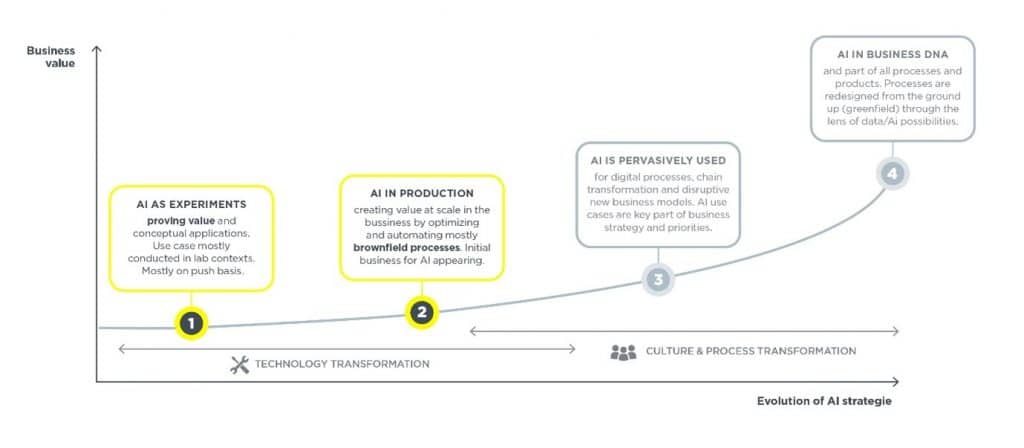
More companies work with live AI implementations and AI is becoming more advanced
When accounting for the Gartner AI Maturity Model (Figure 1) and considering this year’s results from the DDMA AI maturity test, we see that generally, larger companies (500+ employees) are further along the AI adoption process than smaller ones. Moreover, 27% of participants in the survey indicated that they have live AI implementations. That’s a 17% increase compared to 2021. This implies that the majority of companies are still in phase 1 or have advanced to phase 2. Furthermore, a positive trend is that the distance between AI and business teams has decreased. Now, more than half (57%) of the companies state that both groups frequently or very frequently collaborate on AI projects. When it comes to working on AI, 84% say they can explain their AI models. However, half of this group points out their focus is more on getting results from AI, rather than on spending time on being able to explain it.
Another important insight that challenges the adoption of AI, is the fact that only 20% of the companies surveyed state that there is a larger overarching organisational vision on scales of the development of AI initiatives. Although there’s room for improvement in terms of vision, the maturity test indicated that utilised AI models have become more advanced in comparison to last year. Now, around 10% mainly use statistical techniques, compared to 50% in 2021. In addition, we’ve seen a year-on-year increase of nearly 60% in the use of more advanced machine learning and deep learning techniques.
An area which also shows potential when it comes to AI adoption is that of processes. Even though 80% of the companies say they use automated pipelines for AI models, within this group, merely 20% employ an ML lifecycle management system. Looking at what AI is used for, some organisations (33%) state that certain relevant business processes make use of AI, while 60% say that AI is used for true innovations and not just gradual improvements. It is interesting to note that AI engineers are more optimistic than those on the operational side about the use of AI for either relevant business processes or innovation. Productionisation of AI remains a challenge. Nevertheless, it is encouraging to observe that both the AI engineering and operational side agree that 10-75% of productionised AI models do have a measurable (positive) impact. Last but not least, there appears to be a correlation between more complex models and a greater impact. However, it is important to note that, in practice, more complex models are generally no guarantee whatsoever of greater impact.
How to get to the next adoption phase?
From phase 1 to phase 2
Looking at the DDMA AI Maturity test, the vast majority of Dutch companies are in phase 1 (AI as experiments) and phase 2 (AI in production) of AI adoption. Specifically for companies in phase 1, you need to be able to answer ‘yes’ to two questions:
- Do you have a good understanding of what AI is?
- Can you identify potential AI use cases within your organisation?
If you can answer ‘no’ to one or both questions, you’ll probably need to consider turning to external AI consultants if you are to acquire the required knowledge quickly. Although this is likely to be costly, you could also consider developing the required skills and knowledge in-house or hiring a data scientist or possibly graduate students.
Moreover, it’s important to think in small steps and in an agile way of working; i.e. start with initiating AI as experiments and proof of concepts. This means that proof of concepts should be pre-evaluated based on factors such as project size, feasibility and potential business value. An ideal proof of concept should be able to deliver real value within half a year. In that light, organisations should start with simple rather than complex AI models. Simple models usually give quicker results that are easier to explain to the rest of the organisation. This will help you get more people from your organisation on board with supporting your AI activities so they become AI evangelists themselves, resulting in greater traction for AI adoption.
How to get to the next adoption phase?
From phase 2 to phase 3
Once AI adoption gets more traction within your organisation and you move into phase 2 (AI in production) you can do the following things:
- It’s time to get more employees working on AI or learning more about its potential impact on your organisation. Preferably, with business and AI colleagues working together in a cross-functional team or teams.
- Don’t forget to spend time on model explainability and try to explain your models in terms of customers if possible; “because of X, customer Y achieved this result”. If you can’t explain a specific model, please make an educated decision to not use it. If you’ve already run some AI experiments, then develop a vision about where you want to go with AI inside your organisation. This will help you acquire more AI funding.
- In the long run, this vision and interdisciplinary collaboration will pay off when identifying valuable new features or services that support your core business. Considering other aspects of AI projects such as legal measures and ethics will help with this too. Knowledge about these aspects should be taught to all your AI experts.
- You should also remain critical when starting or not starting new AI projects by performing predefined checks using AI blueprint checklists. This will help to deliver value faster and get more AI models into production.
- From a technical perspective, you can further enhance the productionisation of AI by setting up an AI Operations environment / ML lifecycle management system that supports the entire workflow for AI projects. This is also known as MLOps, which covers things such as the automation of pipelines, model deployment, model serving results and monitoring. By embracing this approach, AI modelling will no longer be a manual process, it will be fully automated and will continuously deliver incremental value for your organisation.
Please always bear in mind that you should not view the task of leveraging AI as a technological challenge, Make sure you bring the right set of tools and capabilities into the organisation. As we are some years past the initial peak of the AI hype, many companies find that by doing this they haven’t reaped the benefits they initially planned to.
So, there are a few tips to help you advance from the first two stages of the Gartner AI Maturity Model. Below you’ll find an overview of the phases typical organisations pass through and the tips you can use to advance from one stage to the next.
How to get to the next adoption phase?
Tips & tricks
- AI initiatives are conducted in silos (lab environments)
- Highly experimental
- Mainly push from AI group; no business pull yet
- Small group of highly skilled individuals
Tips on how to advance
- Get a good understanding of what AI really is and identify potential use cases for your organisation
- Develop the skills yourself, find an external consultant and/or hire a data scientist (potentially graduate students)
- Then start working on it :). Start building and make experiments/proofs of concept (keep it simple)
- Include more people in the projects and educate the workforce about what AI is and what it could potentially bring to your organisation. Not only more technical people but also colleagues from the business side. It might help your organisation to hire an external expert to boost your projects
- Start organising your data analysts or data scientists so they can help each other and make more impact. Perhaps train data analysts to become data scientists.
- Find a sponsor in senior management and get some innovation funding to fire up the engines
- Start experimenting with AI to deliver real value
- Choose some smaller and feasible AI pilot projects, and try to get them live in production
- Try to timebox projects, and start on something small that could deliver real value within half a year
- Focus on optimising or automating time-consuming or high-effort supportive tasks where you can bring value that was not possible without AI
- Work on analysing structured data from data dumps or databases. Ideally, you would start organising your data in a central database such that data scientists/analysts have full access to all data
- Start training your models manually (ideally on a regular basis)
- Develop more computer science skills like Git, structured programming, deployment, etc.
- Make sure that data scientists have powerful laptops and can use a departmental server for AI purposes or, if possible, have some budget for cloud AI solutions and/or VMs
- Standardised pipelines (MLOps)
- Mainly push from AI group; business pull slowly taking off
- Usually organised as an AI Centre of Excellence
- Mostly brownfield AI implementations (automating existing processes) instead of greenfield ones (redesigning processes through the lens of AI possibilities)
Tips on how to advance
- Getting more internal employees working on AI or taking the time to learn about the impact of AI
- Let business and science colleagues work together more frequently on AI projects
- See if you can set up data science teams in more business units to spread the knowledge
- Find sponsors in the regular marketing/ IT business lines and have them make budget reservations for AI
- Think about predefined checks at the beginning of a new project and check ethical issues in your validation steps
- Try to explain your models on a customer level; “because of X, customer Y got this result”. Or when you can’t explain it, take an educated decision to (not) use an algorithm.
- Start considering legal and ethical aspects of AI projects, as well as how you can explain how your models work
- You have run some AI experiments by now, and should be able to grasp the idea behind AI and the potential for your organisation. Develop a vision where you want to go with AI inside your organisation that supports getting funding in place.
- Try breaking AI projects down into smaller steps so you can deliver value faster and get more into production
- Try looking at adding more new features or services that support the core business
- Start more AI projects on a wider range of business processes to spread knowledge and increase business process knowledge among the AI experts
- Start looking into an AI Operations environment supporting the entire workflow for AI projects
- Add more complex data sources like free text, images and audio content to enhance your business
- Explore more complex database systems/file systems such as Hadoop, S3 and distributed database solutions and update your models regularly with those systems
- Start to look into batch and streaming (online) Machine Learning models that can be updated in real-time. Also, see if you can start a proof of concept on deep learning.
- Look into scaling up the on-premise server to a cluster of servers such that models can be trained in parallel
- AI is a key pillar in business strategy, with the strategy being translated to supporting data & AI initiatives
- All personas are engaged in the use case lifecycle (business, AI, data, IT)
- Mostly brownfield AI implementations (automating existing processes) instead of greenfield (redesigning processes through the lens of AI possibilities)
Tips on how to advance
- Make sure the value of AI is appreciated from the work floor to board level. Also, get as many internal employees from a range of backgrounds to learn about AI or be part of projects that include it
- Get all layers of the organisation involved. Include higher management so heavily that they will initiate AI projects themselves
- Make sure business and data scientists/engineers always work together as a multi-disciplinary team on an AI project
- Arrange budgets for your projects, but on top of that find a budget specifically to develop new capabilities for future value creation.
- Incorporate legal and ethical aspects and involve colleagues at every stage from start to finish. Consider using a legal and ethical board to review AI activities.
- Challenge your teams to explain your AI models at a customer level externally or estimate as well as possible.
- Use AI to radically innovate and improve your core business or develop new products or services that were not possible before
- Try getting more of your pilot projects towards the actual production phase, where they go on to deliver consistent business value
- Try looking at project organisation and input before a project starts, so you are in a better position to use timebox projects and deliver value even faster
- Find ways to easily deploy and automatically update your AI models on top of cutting-edge solutions like S3, Hadoop or distributed database solutions
- Start to look into Deep Learning models to boost the performance of your AI stack
- Consider moving to a cloud solution such that your AI services easily scale (also using hosted services involves much less maintenance)
- Processes are redesigned from the ground up (greenfield) through the lens of AI possibilities
- New products and services are unlocked based on AI possibilities
Tips to consider
- You are on top of your game! Based on your answers, it seems you are at the highest level. Now it is important to remain at that level and perhaps upgrade some of the aspects that still need improvement. If you haven’t done so yet, this might also be the time to start sharing your knowledge and experience with other organisations. Together we can do greater things with data and AI!
Ook interessant
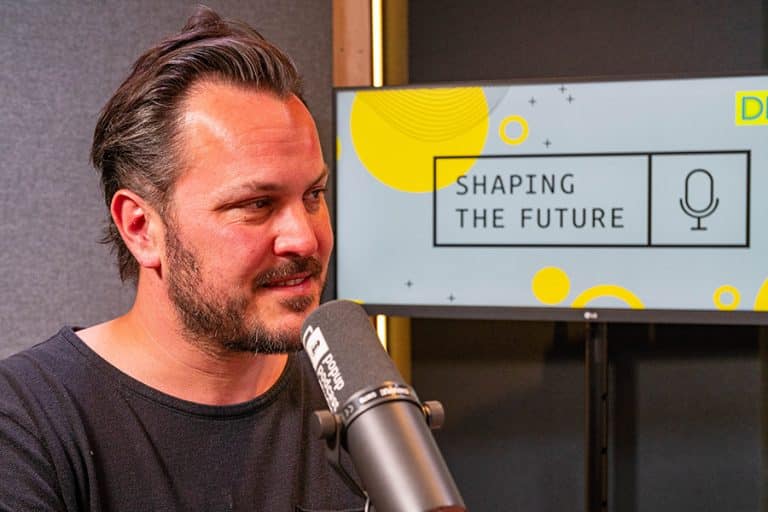
AI in marketing: van praten naar doen – hoe je écht aan de slag gaat
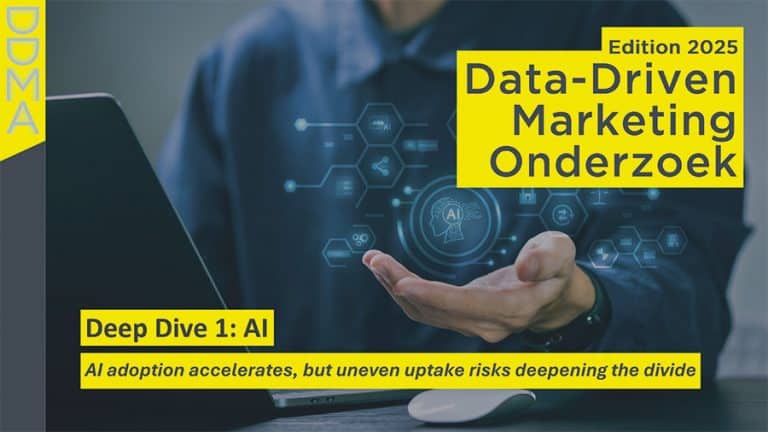
DDMO 2025: AI adoption accelerates, but uneven uptake risks deepening the divide
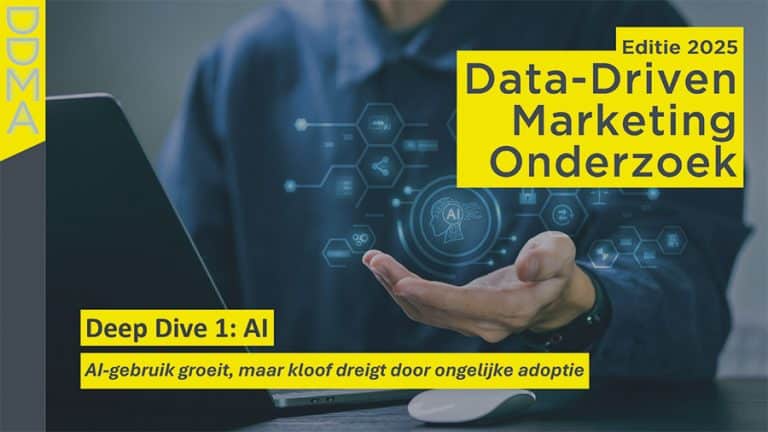