What is your role at Randstad Group Netherlands?
I am Lead Data Scientist and Chapter Lead at Randstad Groep Nederland. This means that I oversee all data scientists in the different Agile teams within our organization, active in IT, HR, Finance and Marketing Communications, among others. All those data scientists together are intertwined in our AI chapter. From here, I make sure we’re all pointing the same direction and that we are not doing double work. Which is quite a development looking at our history. We have shown that we’re good at bringing complex AI systems into production. But until a few years ago, we’ve not been very good at keeping each other informed. The chapter structure ensures that we do now.’
What road have you travelled in search of a mature deployment of AI?
In terms of maturity in the field of AI, I feel we are doing quite well at the moment. This feeling has been validated from the outcome of the DDMA AI Maturity Test. We score a 3 out of 4. Like many organizations, we started with AI from the angle of innovation. We saw what was happening with AI in the field of technology and science. And based on that, we started our first use case: AI Matching, which means we started developing “Recommender systems” like the ones we know from Netflix and Amazon. We also wanted to make recommendations, but in the field of human resources. We wanted to show job seekers relevant jobs based on AI, which turned out successful! Since then, AI has become part of Randstad Groep Nederland much more widely and we deploy AI to get value out of data in the broadest sense, which increased our AI Maturity tremendously.
How do you create support for AI within your own organization? Especially if you’re just starting out?
Developing AI goes hand in hand with evaluating its impact. With measurability, the first way to create support in the organization is to be able to show the impact AI can have. In addition, it is crucial that you engage with all stakeholders within your organization. AI used to be only an IT game; you would experiment with models and be happy if something went right. Now you really have to take a broader approach. For example, we are in daily conversations with spokespeople, privacy officers, our diversity & inclusion task force and other stakeholders. We formulated our own AI principles, and established a constant contact to discuss the possible implications of AI systems. This conversation is a very important prerequisite for creating more support.
When it comes to AI, people often see many bumps in the road; it costs money, finding good people is difficult, etc. Is this also a challenge for you guys?
Finding good people is always a challenge. Our large academic network has helped a lot with this. Because people in academic circles are often at the forefront of AI, that is also where most of the talent comes from. We therefore spend a lot of time getting involved in the academic world by publishing papers and collaborating with academia in various ways, and found that resonates very well with job seekers who have just graduated in the field of AI. After all, they prefer to work for companies that are leading the way in AI.
In addition, it is also very important to keep up with the technical side of things. For example, we are working on a major renovation of our data lake. This is an important development for us, because it enables us to develop better AI applications faster and easier, but also because it makes us an attractive employer for AI talent.
For a lot of people AI sounds complicated. Where exactly should you start as a company just starting out with AI?
You can start by consulting an AI consultancy. They can come up with an interesting first use case. From there, you can look at whether you want to have ownership of your AI applications and whether you are willing to start investing in them. There are also many off-the-shelf use cases available. In the marketing domain, for example, a commonly seen use-case is churn prediction, to predict if and when customers or subscribers might drop out of your product or service.
How can the DDMA AI Maturity test help organizations?
First and foremost, the DDMA AI Maturity Test is a very useful benchmark. It gives you an idea of where you are and where you can potentially go with AI. The question of where you can go is actually concretely answered by doing the test, because you get practical pointers to take the next steps towards maturity. In addition, it gives you an opportunity to reflect. And that’s good for every organization. Where are you at with AI? Is this what you envisioned? Do you want to continue working from here or are fine with where you are?
There currently is a lot of discussion about the explainability of AI models. Can you still make consumers or even organizations understand what is happening with their data? How do you make sure you stay compliant? After all, you are working with off-the-shelf algorithms and advanced platforms and techniques.
For us at Randstad, compliance with respect to security and privacy is a top priority. However, part of staying compliant in the future is still a matter of speculation, because in terms of legislation we do not yet know exactly what we will have to comply with. But by definition, AI will definitely evolve alongside legal developments. Both organizations that develop AI applications and cloud providers will have to make sure that their products or services are compliant. Only the companies that are currently far along with developing AI themselves may have a lot of work ahead of them to retrospectively make sure everything is compliant. In short, compliance in AI right now goes hand in hand with the development of the whole AI field.
Ethics play a central role in AI development. That’s why you need to make sure that data scientists or AI developers are not working in isolation. Reasoning about and developing AI products should not purely come from the perspective of technology, but is a multistakeholder endeavour. To guarantee this, these earlier mentioned conversations with different stakeholders are so important. In addition, we have formulated our own AI principles that serve as guidelines for AI development. In the end, you have to work from the values of your organization,and the customers you serve. You really have to take up a company-wide approach for successfully leveraging the value of AI.
How do you see AI developing within the data-driven marketing sector in the coming years?
On the technology front, you see the rise of “AI democratization,” where AI technologies are becoming much more accessible to anyone, not just the major technology companies. Cloud providers are providing ready-to-use components, and platforms are providing means to quickly tap into your own data in order to quickly develop AI prototypes. In addition, there will be more off-the-shelf use cases.
Finally, there is also a lot happening in the legal field. There is more legislation heading towards us and developments like the cookieless world will have their impact. All in all, this means that as an AI developer you need to be well aware of how you deal with data and what you use it for. In addition, it is crucial to be flexible and remain adaptive. You need to be able to constantly adapt to new developments, whether legal, technical or social.
Are you inspired by Davids story? Do you want to know how far along your are with your AI Maturity and are you ready to take the next step? Then take the DDMA Maturity Test and receive hands-on recommendations to improve your AI maturity. Take the test here.
Ook interessant
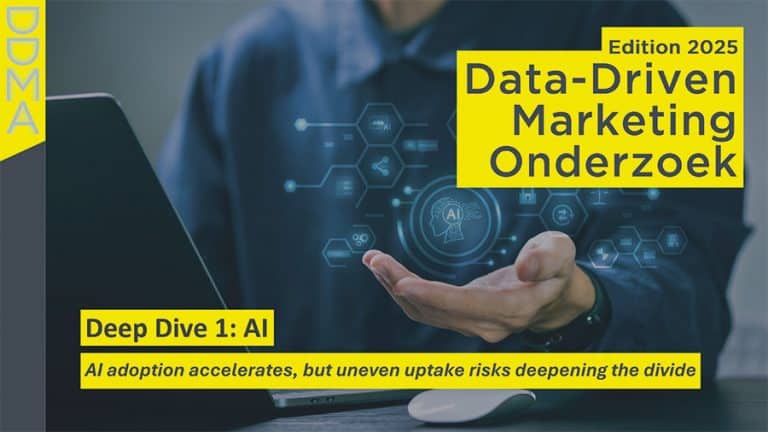
DDMO 2025: AI adoption accelerates, but uneven uptake risks deepening the divide
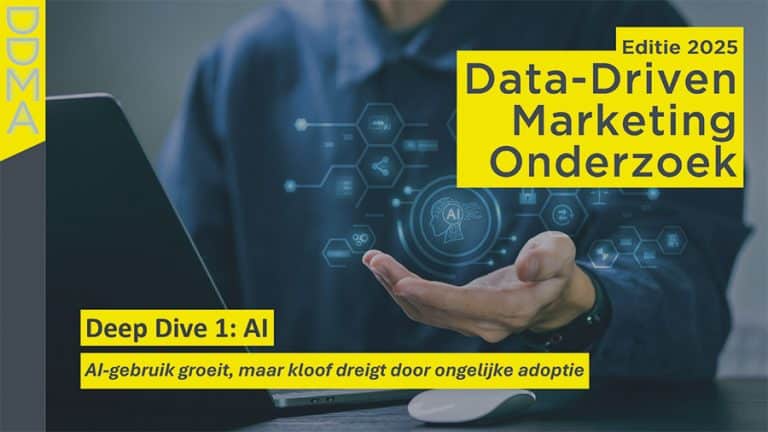
DDMO 2025: AI-gebruik groeit, maar kloof dreigt door ongelijke adoptie
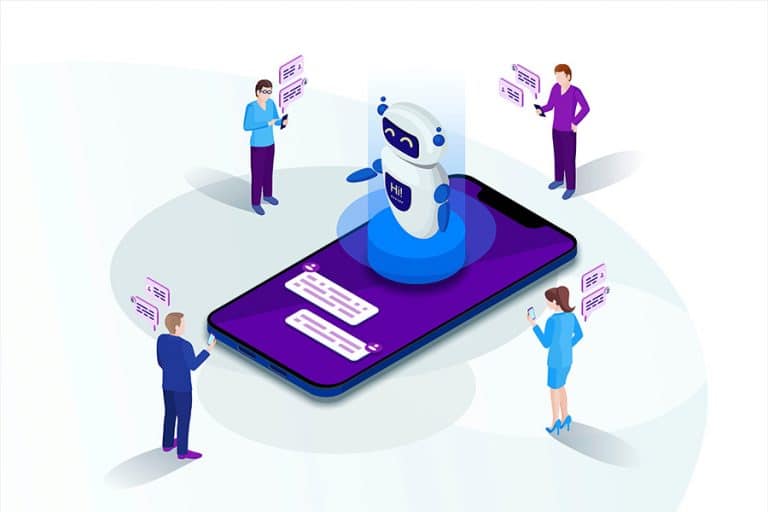