Great examples of Data Science often emanate from companies that have already come a long way in developing their use of data. Although for some organisations, such examples are highly relevant and directly applicable, this is not true for all of them. In this article, taken from the DDMA committee on Data, Decisions and Engagement, we describe how you can create business impact in the different stages of developing a data strategy, using several basic principles and the ‘’people, process & technology’ model.
Three basic principles from which to start using data professionally
Regardless of the maturity of your organisation’s data use, there are three basic principles that can help you use data professionally. As your organisation matures, these principles are applied in a different way.
- Place the business challenge centre stage; a data team has to solve issues that have a business impact, which is why it works very closely with the business. If it doesn’t, it will become detached from the business and its added value will be diminished. Rather than focusing exclusively on optimising a model, be sure always to keep sight of the overall goal: the impact of the model on your organisation’s sales.
- Take small steps that quickly create value for the business. Companies that are just getting started with using data can develop simple data products, such as a clear dashboard with an overview of their main KPIs. It is also important that more advanced companies carry on delivering value and celebrating successes.
- A sponsor at MT level, who removes objections and promotes a vision that emphasises the importance of using data in a smart way. Every data team needs this support because data projects tend to stir up resistance. Employees might, for example, be afraid that their jobs might be taken over by computers, or they might feel that collecting, unlocking and analysing data could take up a lot of time.
People, process & technology
The ‘people, process & technology’ model is a handy tool for clearly identifying organisational changes. By examining three aspects – people, process & technology – of Data-Science projects, you can make sure improvements are carried through efficiently.
1. People – Make sure you don’t lose sight of the business with Data Science
Organisations that use Data Science usually don’t start out with a large data team; rather, they build it up over a number of phases. The professional use of data in companies often starts with data analysts who are on business teams, such as the marketing team. The analyst is fully involved and works constantly on solving business issues, for example by developing dashboards or analysing sales or customer retention. The next phase sees many companies setting up separate data teams. Although there are many advantages to this, such as the various business teams using the same definitions and technology and sharing knowledge, it also has presents a potential drawback: distancing from the core business. In such a setup, you need to make sure you have a number of forward-thinking data people on your business teams. Organisations that develop and start working on setting up advanced analytical models also make sure that there are people who can work on the model for longer periods, often six to twelve months, without being distracted by the daily turmoil of business. At that stage of maturity, there are often specific positions designed to provide a link between the business and the Data Scientists.
2. Process- A data project from Discovery to Deployment
The process for a data project consists of several steps that can vary greatly in complexity, depending on the number of people working on the project and the maturity of the organisation. Experience shows that these phases are completed more explicitly and take more time with larger organisations and more complex projects. Agile working is preferred so that constant adjustments can be made in short cycles. The steps in a data project consist of:
Discovery
The first step in a data project is to formulate a clear goal. This is done by collecting the needs of the business. This is what is known as the discovery phase, in which you determine an MVP(Minimal Viable Product) and scope during an interactive session with the key stakeholders. This phase also looks at what data and models are already available to answer the business question, and what still needs to be developed.
Alignment
The alignment phase formulates the definition of success, together with hypotheses and a schedule. In this phase, you create a stakeholder map showing who the main stakeholders are and who makes the decisions? To maintain support and to make any adjustments in line with their wishes, they are continually consulted.
Data preparation
The next phase is data preparation. During this phase, data scientists browse the data to identify the data structure and the most valuable data. They also validate the data and remove the outliers. This is done in consultation with the main stakeholder. While validating the data, it is often handy to perform a simple correlation analysis so you can recognise and explain the relationships between different variables.
Modelling
In the modelling phase, a model is built. With all the software available today, this is not even that complicated any more. However, it is important to look at the different types of models and which one best suits the business purpose. Examples of models are:
- Classification model, for calculating a Next Best Offer
- Clustering model, for customer segmentation
- Forecast model, for pricing models
- Time series model, for predicting calls to the service centre
- Recommender model (collaborative filtering), to promote products based on interest shown (‘who bought this, also bought…’)
- Outliers model, for fraud detection
Depending on the model, you can examine various statistical and other values to test the quality and predictive value of the model; for example, Accuracy, Confusion matrix, Root Mean Squared Error, Gain and Lift charts, and R-squared.
Evaluation and coordination with stakeholders are also very important during this phase if you are to maintain focus on the end result and the business impact. Data scientists can sometimes get rather caught up in the latest technologies and performance optimisation, thereby losing sight of the overall goal.
Test
In this phase, you combine the intelligence of the model with the knowledge from the business to create a test plan. This allows you to test whether a model is capable of generating business impact because models on their own won’t produce any results. No matter how well you can predict which customers are about to cancel, it doesn’t help you prevent them from doing so. A test plan lets you measure and improve the effectiveness of the model for the business application. Ideally, you test a model with four different groups: two control groups (one with the model and one completely randomly) and two campaign groups (one with suggested action and one without). By comparing these four target audiences and the results, you can assess the model’s performance and its effectiveness for the application (e.g. a campaign).
Deployment
If the results in the test phase are good, the final step is to automate the model. In the deployment phase, you put the model into production. The data flows are automated, the model runs periodically and the results are fed back to the business teams, who then act upon them.
As previously mentioned, whether you complete these phases gradually depends on the organisation’s maturity in terms of Data Science. An individual analyst in a marketing team will not necessarily go through each one of these steps when building a dashboard. However, in larger organisations where developing complex models happens more frequently, every process step is crucial.
3. Technology – Data Science is becoming easier and more accessible
When it comes to technology, we are seeing a number of developments. First of all, Data Science is getting easier thanks to the software available. With “no-code” tooling such as Rapid Miner, Dataiku or KNIME, you can prepare data, test models, optimise, compare results and put the best model into production without any data or programming experience. However, you’ll still need knowledge of data structures, statistics and the logic behind these types of models. If you don’t understand what is happening under the bonnet or cannot interpret the results properly, you can easily get things wrong by, say, “overfitting” models. Besides being easier, Data Science is also becoming more accessible, thanks to the integration of Data Science functionalities within cloud environments, such as AWS Personalize, Google Recommendations AI and Azure GPT3, and marketing suites such as Salesforce and Selligent
As Data Science becomes seemingly easier and more accessible, it may give rise to the idea that soon every marketer can click together predictive models, almost like creating a PowerPoint presentation.We don’t expect this to be the case though. Data Science knowledge will continue to be necessary for the development of reliable and robust data applications, and for the proper completion of the steps set out above. Nevertheless, the role of a Data Scientist may well be changing. The emphasis for the Data Scientist will shift away from writing the code for the model to exploring the possibilities data has to offer, guiding the process, sparring with the business and determining which model can best be deployed to achieve the business goal. More often, the Data Scientist will switch off his or her screen and go for a walk with marketers, controllers, managers or other specialists from the business. The Data Scientist’s job was already a sexy one, according to Thomas Davenport but it may become irresistible in future.
Key takeaways
More and more possibilities are opening up in the field of Data Science, and harnessing it is becoming easier and more accessible. For many organisations, this means there are opportunities that didn’t previously exist. We hope that this article will help a wide variety of organisations to effectively set up their Data Science activities and provide the tools to achieve business results through the smart use of data. We have listed the key takeaways below:
- You will have a business impact with Data Science if you continually focus on the end result: the business application of models or other data products
- For any organisation working professionally with data, a few basic principles apply: place the business challenge centre stage, take small iterative steps and find a sponsor, preferably at MT level
- Rather than starting out with a large data team, companies usually start with analysts in business teams. They often evolve into specialised data teams with roles that bridge the gap to the business.
- The process of data projects comprises several phases. The business is involved in every phase. Whether and how organisations complete every one of the phases in practice depends on the organisation’s maturity and the complexity of the data product.
- Data Science is becoming easier and more accessible. Although the role of Data Scientists may be changing, it remains as important as ever.
Ook interessant
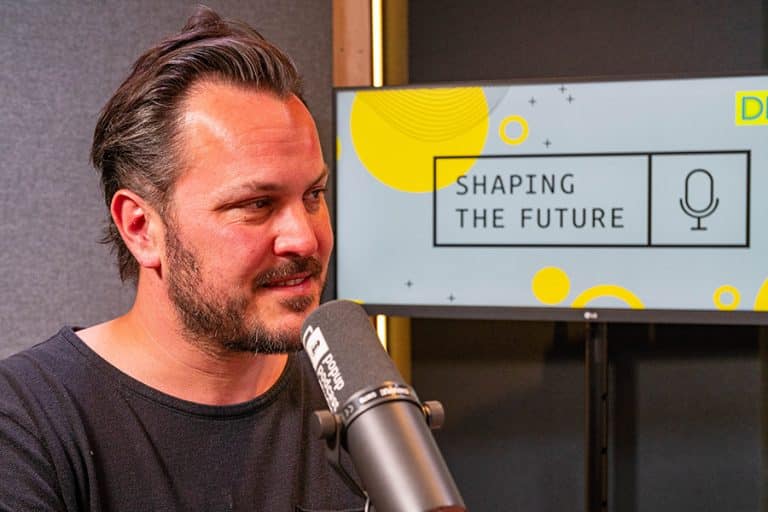
AI in marketing: van praten naar doen – hoe je écht aan de slag gaat
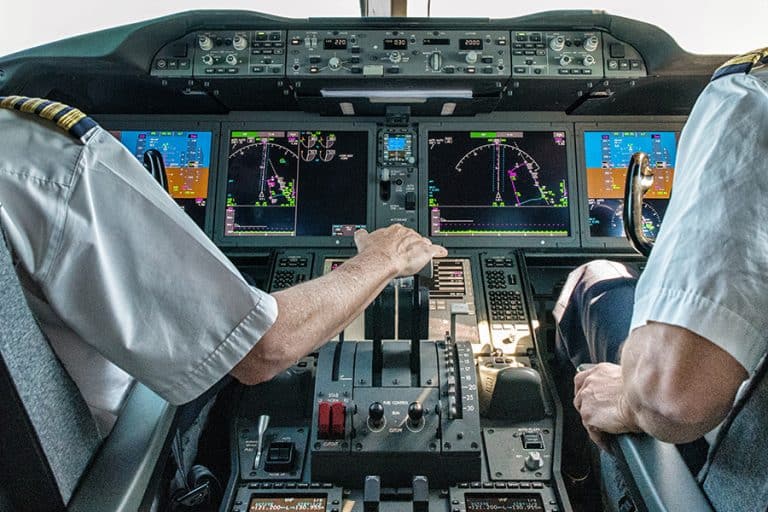
Media Effectiviteits Meting (MEM): Essentieel voor Efficiënte Groei
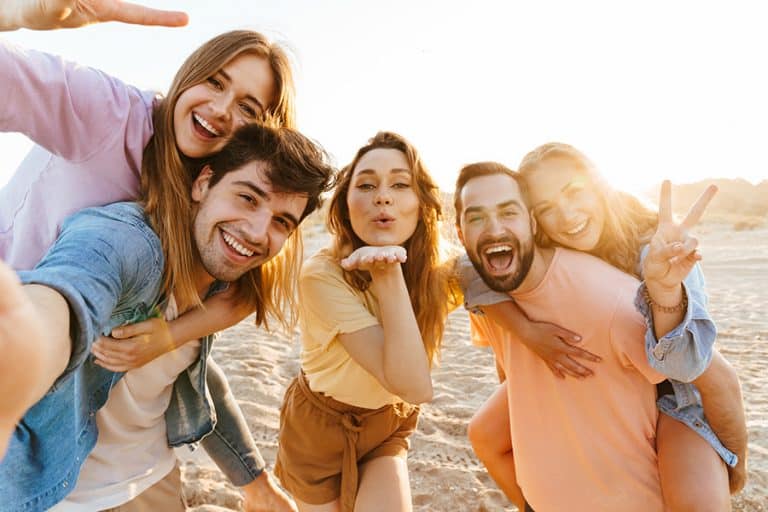